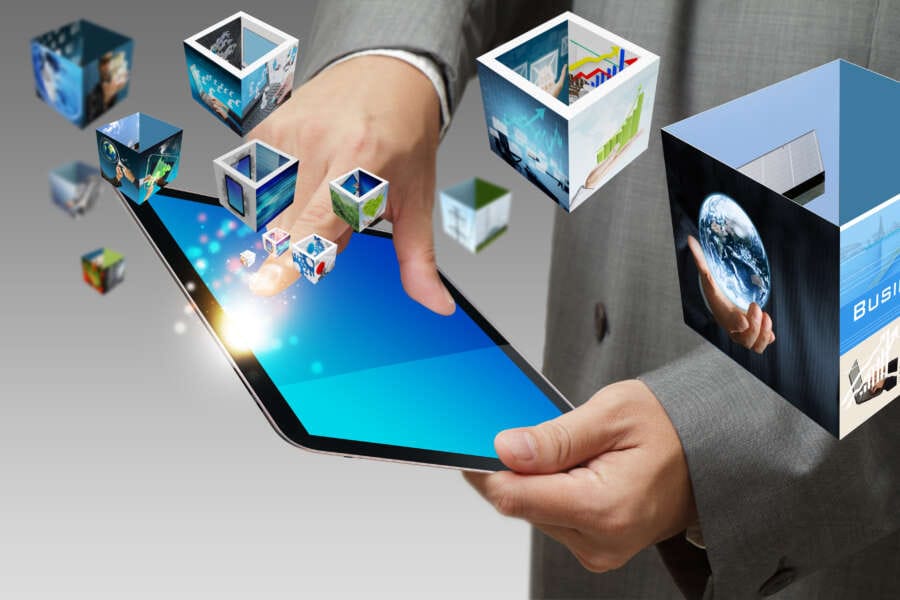
The latest release of Canonical’s end-to-end MLOps platform brings advanced AI/ML training capabilities
8 September 2022- Canonical, the publisher of Ubuntu, announces today the release of Charmed Kubeflow 1.6, an end-to-end MLOps platform with optimised complex model training capabilities. Charmed Kubeflow is Canonical’s enterprise-ready distribution of Kubeflow, an open-source machine learning toolkit designed for use with Kubernetes.
Charmed Kubeflow 1.6 follows the same release cadence as the Kubeflow upstream project. This latest version follows the Kubeflow roadmap and comes with performance enhancements and more advanced model training capabilities.
Speed up digital transformation: take models from concept to production
Charmed Kubeflow helps data science teams automate tasks and boost productivity, helping companies lower costs. The platform’s components use charms – Kubernetes operators that automate maintenance and security operations. Charms accelerate workload deployment, allowing data scientists to take models to market more efficiently.
According to a 2022 IBM Index AI Report, adoption of AI/ML within enterprises reached 35% last year. The benefits are clear. For instance, banks applying ML to build recommendation earnings have boosted sales by 10%, saved 20% in CAPEX, increased cash collections by 20%, and reduced churn by 20%. Identifying tools that bring automation to data science workflows has become essential to getting a faster return on investment from AI/ML projects.
Improved data processing and tracking with MLOps
Besides accelerating deployments, Charmed Kubeflow 1.6 makes data processing more seamless. Kubeflow’s 2022 survey reveals that data processing and transformation are the enterprise’s most challenging and time-consuming activities. Moreover, data comes from various sources, each with particular processes and dependencies.
Charmed Kubeflow 1.6 comes with better tracking capabilities. AI/ML models can be measured more effectively; evolution and debugging become simpler. The solution detects data drift and allows models to adapt to it quickly. Charmed Kubeflow 1.6 brings improved tracking for trial logs as well, allowing for efficient debugging in case of data source failure.
Optimised AI training and modelling
Models need up to 15 iterations to become production-ready, and only half of them get that far into the AI/ML journey. Charmed Kubeflow 1.6 supports population-based training (PBT), accelerating model iteration and improving the likelihood that models will reach production readiness. An MPI operator makes training large volumes of data more efficient. PyTorch elastic training enhancements make model training more effective and help ML engineers get started quickly.